7 Challenges of Adopting Artificial Intelligence Solutions
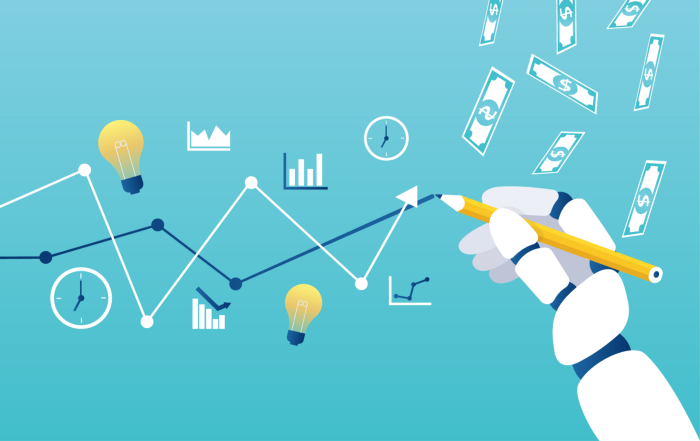
By Adnan Kharuf
Artificial Intelligence has begun providing real value to organizations in various industries. This will start becoming more apparent in the future as AI solutions become more accessible and easier to implement. However, even with the high levels of interest in leveraging AI and ML solutions, implementation and deployment in many organizations is still low. Much of this is because enterprises don’t incorporate some realities about AI projects into their thinking.
Let’s explore seven challenges that enterprises need to overcome to successfully leverage AI solutions.
Business Case
Organizations need to understand and identify the clear problems that utilizing AI and ML solutions will potentially solve. They will need to think about how they can add AI capabilities to existing and future processes, products and services. Instead of simply painting a broad vision, they need to also be clear on what impact it will have on the business over the short and long term.
Very often predicting and measuring the returns on investment in AI is difficult, especially when the results are not apparent right away. But in the long run, the built-in continuous learning that comes with AI will help organizations adapt to changing business conditions in a more flexible manner.
Skills
Organizations need to hire specialists who have deep knowledge of current AI technologies and their limitations. Additionally, it is necessary to supplement these AI specialists with subject matter experts that can provide context and clarity to business problems. The experts also need a complete understanding of the organization’s business goals and technology needs to help implement an AI strategy. Organizations with a limited budget may not be in the position to hire the right talent that is required for an AI project. It will take a lot of time and work for organizations to find well-trained professionals with the right skill sets who can build an enterprise’s AI solutions from the ground up.
Cost
AI/ML experts, business analysts, data scientists, and subject matter experts in today’s market are hard to find and expensive. Developing, deploying, and maintaining an AI solution from scratch will require data engineers, software engineers, product/project managers, ML/AI experts and the right infrastructure. The total expense that is required for a typical AI project is often quite large, and doing one-off projects are especially expensive.
Tools
According to Gartner, a team that’s building data science algorithms and solutions uses seven tools on average to build a solution. Many decide to build their own AI solution by combining multiple data processing (Spark, Hive, etc.) and AI/ML tools (Spark ML, PyTorch, TensorFlow, etc.). Many of these tools are rapidly evolving open source applications that are inadequately integrated across end-to-end data workflows. Not only will this limitation slow down innovation but will also create large security and process vulnerabilities.
Data
AI solutions are built and driven by data. Organizations must have base data as well as a constant source of data to keep it up and running. But AI is also dependent on the right kind of data, not just any data. It remains challenging for organizations to integrate data since information is usually spread across multiple applications in various formats such as text, image, video, and audio. Experts know success with AI will depend on quality data to build models and provide accurate learning and results. For example, in certain industries such as healthcare, it is difficult to predict outcomes of breast cancer if there is a lack of patient data sets to ingest. Organizations need to consider the problems AI will need to solve and take the time to prepare the data.
Infrastructure
Data handling, storage, compute, scaling, extensibility and security are all critical components necessary for enterprises to deploy an AI solution. An organization’s ultimate success with AI always starts with how suitable its infrastructure environment is to support powerful AI applications and workloads. This includes:
- The right mix of processing capabilities and high-speed storage to support state-of-the-art machine learning and deep learning models.
- The right software that is tuned and optimized to fit the underlying hardware.
- A single interface that can manage most moving parts and components.
- A flexible infrastructure that can be deployed in the cloud, or in an on-premise data center to optimize performance.
Organizations need to think about infrastructure more broadly to successfully enable AI today and in the future.
Integrations
Ultimately, the success of AI implementation within an organization will depend on how well the solution integrates with existing infrastructure and business functions. An organization needs to be flexible enough to adapt new business models, new team models, and new workflows across all departments and teams. Incorporating AI into the business is as much a people and process problem as it is a technology one.
Conclusion
Once an enterprise organization can overcome these challenges, they will finally be able to utilize AI to drastically revolutionize businesses, improve processes, and increase employee productivity. The key will be to minimize challenges and maximize the benefits to adopt the core capabilities of AI. Organizations will need to look for the right approach to enable AI-driven solutions without the need to build everything from scratch. Reusability of components such as data, models, and processing techniques is critical for expanding the use of AI.
Originally published at petuum.com on April 2, 2019.