Petuum Presents At Brain X Community Live
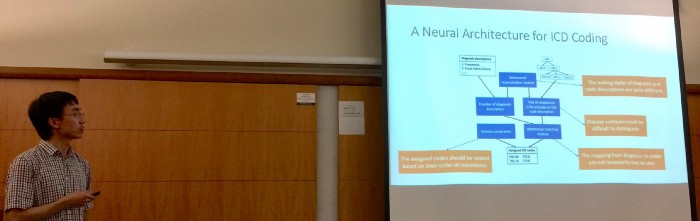
At the BrainX Community Live event at Cleveland Clinic Monday evening, Petuum’s Director of Data Services and Solutions, Pengtao Xie, described his team’s work in applying advanced deep learning algorithms to a particularly tricky challenge in healthcare, extracting critical information from unstructured data in medical records.
His talk focused on findings from a recent research paper titled, “Effective Use of Bidirectional Language Modeling for Biomedical Named Entity Recognition.” Pengtao and fellow authors Devendra Singh Sachan and Petuum CEO Eric P. Xing explored ways to identify and tag medical entities in text using Deep Learning and Natural Language Processing (NLP) models. This process of identifying and tagging entities is known as Named Entity Recognition (NER), and is a non-trivial task, especially in the medical domain.
NER is a widely studied task in NLP research and there have been many attempts to effectively apply NER systems to medical records. However, healthcare is an especially challenging area for NER due to high linguistic variation in medical record data, such as ambiguous abbreviations, synonyms, and jargon in medical terminology.
Often, medical records such as physician notes and patient intake forms are full of context- and hospital-specific terms, for example, the abbreviation “CAD” might be used to refer to “coronary artery disease” and the term “myocardial infarction” is used instead of “heart attack”. A simple dictionary-based approach to NER with exact matching will fail to correctly tag these entities in texts.
Additionally, many clinical texts might include entity names that lack necessary details, for example, a disease might be described as “leukemia” without specification of the form of leukemia, which could be “lymphoblastic,” “null-cell,” “lymphoid,” etc.
The vocabulary of biomedical entities is also especially dynamic — it is ever-evolving and increasing with new discoveries and medical progress — which makes the task of entity identification even more complex and error-prone.
State of the art machine learning approaches for NER tasks rely on high-quality labeled data, but due to the nature of medical record data input, most data is unlabeled and unstructured. There is therefore a need for NER approaches that can utilize easily accessible, unlabeled data to improve the performance of their supervised variants.
Pengtao and the Petuum team’s novel approach to this long standing problem in healthcare has enabled the effective and efficient extraction of critical information from complex medical reports. You can find a more detailed description of the paper in a previous blog post, here.
The implications of this research have many applications in healthcare, such as enabling automated report generation (like discharge reports, which we’ve mentioned in this blog post) and helping physicians locate relevant record data more quickly when defining patient treatment plans. Healthcare practitioners spend increasing amounts of time in front of computers creating and filling out reports, reviewing reports, and searching for the information they need, which reduces the amount of time they are able to spend with patients and on research. By deploying AI solutions that use NER to automatically extract the information physicians need from medical reports, Petuum hopes to give healthcare practitioners back some of that valuable time.
Pengtao’s presentation led to a vibrant dialogue at the BrainX Community Live event, and we’re glad our work is instigating thought-provoking discussions in medical circles like the BrainX Community, a group of over 400 experts in machine learning, healthcare, and innovation. By working with companies like Petuum, BrainX aims to foster and create the next generation of AI applications for healthcare to improve delivery, remove inefficiencies, decrease cost, and enhance the patient experience.