Getting to Know Mr. Zhiting Hu

Our team at Petuum is made up of incredibly talented people. This series will feature the engineers, managers, and creators that keep our company moving forward and make us proud of the work we do together.
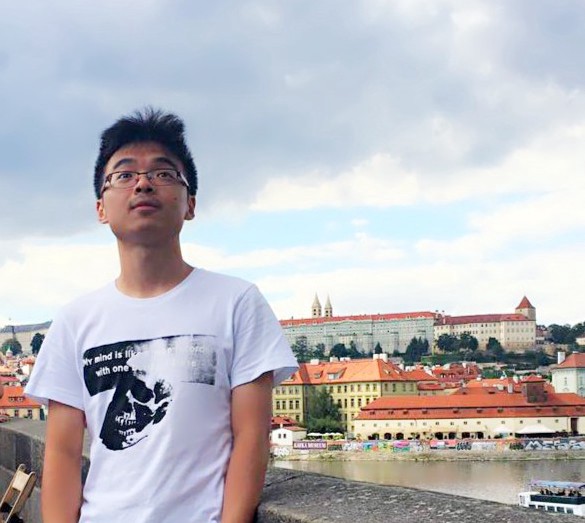
Zhiting Hu is a research scientist at Petuum, Inc., and a senior Ph.D. student in the Machine Learning Department at Carnegie Mellon University. His research is focused on developing a unified learning framework by integrating human knowledge with deep learning, Bayesian modeling and inference, large-scale machine learning, and the applications of these techniques in text generation, natural language processing, and computer vision.
His work on harnessing deep neural networks with logic rules was selected as the outstanding paper at ACL2016. Zhiting is a recipient of the 2017 IBM Ph.D. Fellowship Award and the 2017 Baidu Scholarship. He also won the Excellence Award of Internship in Microsoft Research Asia and the Google Excellence Scholarship.
Why did you join Petuum?
Petuum’s goal is to democratize AI techniques for everyday use and industry use. My personal goal is similar — I want to develop new algorithms that will make artificial intelligence more accessible and practical for people with non-technical backgrounds. The research I’m engaged in as a Ph.D. student at CMU fits well with the work Petuum is doing, so it’s a natural place for me to work and continue to build toward these goals.
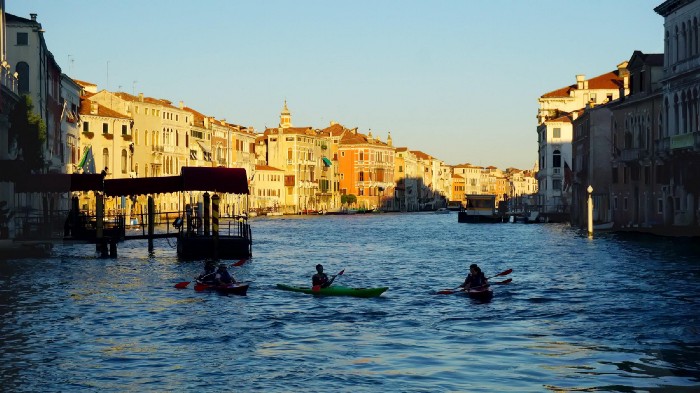
What do you do at Petuum?
I’m a research scientist, which means I’m responsible for research projects and developing parts of our product. I’m currently working on two very exciting projects on natural language processing (NLP) and deep learning. We are taking the cutting-edge of these fields and working on developing completely new theory and technology.
I recently wrote a blog post on how different approaches to deep learning can be unified into a framework that allows researchers to make novel decisions when building models. There are many different types of learning paradigms, but historically, the field has not observed any formal connections between them, so models typically need to be completely rewritten when switching between techniques. We’ve found common properties between many traditional paradigms and designed an interface that allows users to easily flip between them by just changing a few lines of code. This allows for much more experimentation and discovery than we normally see.

What’s the most exciting project you’ve worked on at Petuum?
The NLP work I’m doing now is helping to build a general-purpose text generation toolbox. It will allow users to apply their subject matter expertise to an AI process without needing expertise in the underlying AI techniques. For instance, a doctor could easily use the toolbox to construct a program that automatically generates written reports based off of medical images. This toolbox will be an important component of our products and it’s also a crucial aspect of making advanced AI technologies accessible to everyone.

What’s been most challenging in your work so far?
Because the toolbox needs to be general-purpose, we need to build a set of highly reusable and widely applicable components. To do that, we have to abstract the common properties of text generation tasks, which requires a very deep understanding of the field. I’ve been spending a lot of time combing through the literature to design and structure the toolbox components for optimal usability.

Do you have any advice for people interested in pursuing similar work?
It’s important to not only focus on learning newer techniques like deep learning, but also to spend time researching traditional theory and methods like Bayesian and other canonical techniques. I believe that if people can continue to discover connections between traditional and new methods of machine learning, we’ll all have a better understanding of it and be able to create even more exciting and important technologies.
What do you love to do outside of work?
I enjoy sports like ping pong and swimming and I often play basketball with friends. I also love traveling and photography — I try to do at least one trip to a sightseeing destination every year. Last year I went to Canada and Australia and this year I plan to visit Canada again for a conference in Vancouver.
We’re hiring! We’re growing quickly and looking for highly trained and talented technical staff, including engineers, architects, and more. Contact us at careers@petuum.com.